Introduction
There’s a good chance that data underpins every product, service, or capability in your organisation.
But organisations have been managing data for decades, so why do we need data governance?
At Oakland, we’ve been delivering data governance initiatives to some of the UK’s leading organisations for years.
The primary goal of data governance is to ensure that data assets are managed in a way that aligns with business goals, regulatory requirements, and industry best practices. These extra pressures and demands drive the need to go beyond traditional data management.
We have created this guide to provide you with the practical and pragmatic advice you need to elevate your data governance activities from something that is often done begrudgingly to something everyone in your organisation can get behind.
We’ll talk you through the different factors that must come into play to ensure your data serves its purpose effectively.
And we’ll touch upon the ubiquitous Generative AI shaking the world of DG. It can transform how you handle data, making your operations smarter, faster, and more efficient. Whether you’re looking to automate tasks, enhance decision-making, or innovate your products and services, Gen AI offers an opportunity to unlock these opportunities.
It is very much a ‘get on board or be left behind’ moment for businesses. But where does one start? Not all organisations are the same, and every business will have nuances in requirements and culture, so off the-shelf solutions are unlikely to be a true fit.
Our guide will help you ask the right questions, whether you are currently building your DG strategy or have already begun implementation and consolidation.
Jeff Gilley
Head of Sales
The Oakland Group
Email: jeff.gilley@weareoakland.com
The importance of people-driven Data Governance
One of the recurring themes we’ve observed is organisations trying to drive Data Governance through the focus on technology.
Attracted by the allure of modern Data Governance tools, organisations are investing in what they perceive as a fast track to data and analytics governance maturity through data cataloguing, lineage and glossary solutions.
The problem is these tools will fail in organisations lacking the foundational data behaviours, culture and core Data Governance capabilities.
When forming your Data Governance initiative, it’s essential to incorporate all facets depicted in the framework below. People, processes, and technology constitute integral components, but their success depends on behaviours and culture.
Success in your governance programs can only be achieved by benchmarking and perpetually enhancing all of these elements.
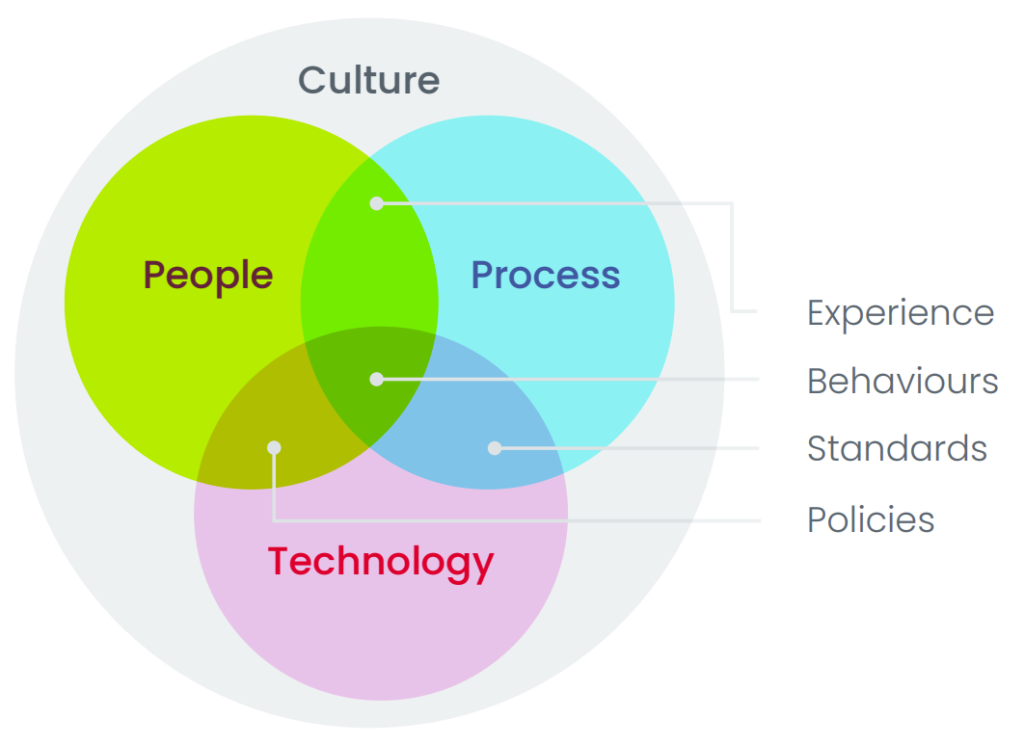
Experience
Beyond knowledge and skills, it’s crucial that your teams grasp how their work contributes to the flow of data across your organisation so they’re able to address data issues. Their familiarity with processes and systems should facilitate easy access to knowledge and tools that help to define and create good-quality data.
People
Data doesn’t magically appear. It demands skilled individuals for its effective creation, curation, and use. Your governance program must prioritise training and education to deliver the required capabilities and knowledge. It’s crucial that they understand the significance of their role and how they support organisational objectives and possess the skills to excel in their tasks.
Processes
Effective processes are crucial for your team to collect, transform, and utilise data. These processes guide data handling, ensuring consistency, quality, and usability throughout its lifecycle. Establishing clear guidelines on data collection, including standardised methods for accuracy and completeness, is a vital aspect of Data Governance process creation. Robust data collection processes minimise errors and inconsistencies, enhancing data reliability.
Behaviours
Changing data behaviours is a gradual process requiring continual effort and commitment to strategic planning, communication, training, and the establishment of effective processes. It involves changing individual habits and transforming the organisational culture to prioritise data quality and effective data management.
Culture
Data culture is both an independent activity and a result of the mentioned interactions. Integral to the Data Governance journey, cultivating a data culture involves managing organisational change and guiding data behaviours from their current state to the desired state. Proactively addressing people, processes, and technology dimensions fosters a culture that transforms data into a valuable organisational asset.
Policies
Effective policies formalise Data Governance best practices, outlining processes for measuring, monitoring, maintaining, and enhancing Data Quality. Policies should also cover how data standards are established, revised and, when necessary, retired. Another critical aspect of policy adoption is defining the roles and responsibilities of key data personnel and stakeholders, ensuring clear lines of responsibility throughout the organisation.
Data Standards
Data standards are guidelines and specifications that define how data should be structured, formatted, and handled within your organisation. These standards ensure data consistency, accuracy, and interoperability across different systems, processes, and applications. Data standards cover various aspects of data management, including naming conventions, data formats, coding schemes, and data classifications.
Technology
Data Governance programs can interact with two types of technology. The first is the everyday technology directly impacting data creation, transformation, and utilisation. The second category is specialised data governance tools that perform various tasks, including documenting data definitions, standards, stewardship, ownership, compliance and movement across the organisation.
The core capabilities of Data Governance
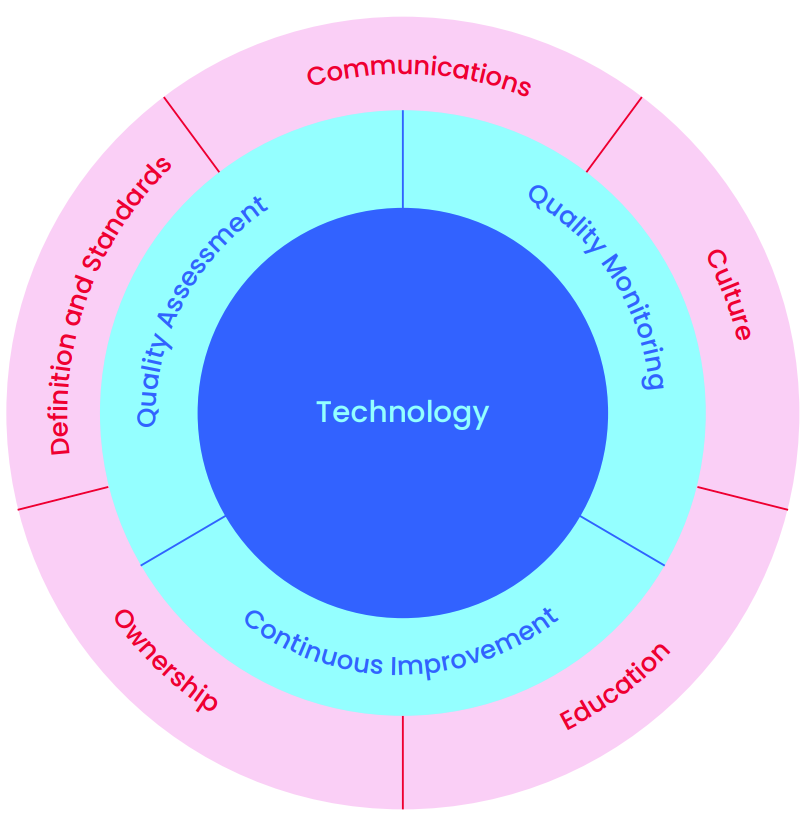
Your Data Governance Strategy
At Oakland, we take a six-step approach to building robust capabilities for your Data Governance Strategy.
Phase 1 – Discovery
- Build a picture of your data landscape, especially your organisational culture and appetite for change.
- Assess your Data Governance maturity to understand how maturity differs across the organisation.
- Evaluate your key stakeholders to understand their current awareness and education levels.
Phase 2 – Education and Training
- Create a communications and training plan for your whole organisation – start small, then build out.
- Develop awareness materials for your whole organisation. This will support further phases by creating a foundational understanding of Data Governance, enabling future stakeholder conversations.
- Education is a continuous part of the Data Governance journey, so consider what training is required at each phase. Staff regularly join and change roles, so engage and educate continuously.
Phase 3 – Roles and Responsibilities
- Define the required governance roles, including accountabilities and responsibilities.
- Engage with all stakeholders to validate the responsibilities and accountabilities assigned to their roles.
- Identify the role holders, assess their training needs, and empower them to undertake new duties.
Phase 4 – Definition
- Collaborate with stakeholders to establish data standards, ensuring that data adheres to them and remains fit for purpose throughout its lifecycle.
- Establish a governance structure to oversee the implementation and adherence to data standards, but opt for an open and inclusive model, never centralised and bureaucratic.
Phase 5 – Assess, Improve and Monitor
- Assess data quality to pinpoint areas of adherence and non-compliance with established standards.
- Focus on improving data quality where it does not meet required standards, ideally through eliminating root causes.
- Facilitate ongoing monitoring and control of data quality, ensuring consistent adherence to data standards and specifications.
- Promote transparency to stakeholders with a shared view of data quality across the entire data lifecycle.
Phase 6 – Technology and Tooling
- Gen AI tools need to be managed as data tools. To make the tool fit for purpose, companies must have rigid oversight on the processes, policies and governance of the data involved (both at ingress and egress) and the tool performance itself.
- Evaluate current and potential technologies that can enhance the management and visibility of data standards, definitions, and quality whilst driving greater automation and continuous improvement.
- Technology is the final piece in your Data Governance puzzle because new tools need the right foundation of culture, capabilities and behaviours to succeed.
Transforming a data culture is not a quick fix – it requires incremental slices of value to be delivered throughout the Data Governance journey, particularly in the earliest phases when you’re moving largely by stealth.
Learn how to launch a successful data governance initiative by delivering rapid value to the business from high-impact data initiatives.
The key to advancing your data culture is identifying the optimal starting point by addressing the greatest areas of pain and reward. To do this, you need a deep understanding of the relative levels of Data Governance maturity across key parts of your organisation.
Let’s explore how to achieve this in the next section.
Discovery
Building a Data Governance Program by Stealth:The Lighthouse Concept | Oakland
Executing a Data Governance Maturity Assessment
A data maturity assessment systematically evaluates your organisation’s ability to manage and leverage data effectively. Its goal is to provide a repeatable framework for evaluating maturity across areas such as data governance, quality, integration, and utilisation.
There are five levels of Data Governance maturity:

Key objectives of a data maturity assessment include:
1. Understanding Current State:
Assessing existing data management practices, policies, and processes.
2. Defining Data Maturity Levels:
Establishing a framework to categorise your organisation’s maturity levels in different aspects of data management.
3. Identifying Strengths and Weaknesses:
Pinpointing areas of strength and areas that need improvement in data management capabilities.
4. Setting Data Maturity Targets:
Defining goals and priorities for enhancing data management practices and moving towards higher maturity levels.
5. Data Strategy Planning:
Informing strategic decisions and investments in data management by aligning with the organisation’s maturity levels and improvement goals.
6. Benchmarking:
Comparing data management practices against industry best practices and standards.
Initiate your Data Governance journey by benchmarking maturity, gaining insights into the current state, and identifying initial steps for a robust foundation. This may include raising stakeholder awareness or addressing critical data set issues. Assessment results will guide key focus areas and priorities for your Data Governance initiatives.
Different business areas or data sets may need varying maturity levels to align with the organisation’s business plans and objectives. Recognising these nuances helps you tailor your Data Governance efforts and allocate resources effectively, ensuring each area reaches the required level of maturity to support broader organisational goals.
Monitoring progress, establishing KPIs, and regularly evaluating achievements and challenges are crucial as your journey advances. This reflective approach enables informed decision-making for refining and enhancing your Data Governance practices.
Promoting awareness and cultivating data maturity among employees is pivotal, with education and communication at the forefront. These initiatives foster a deeper understanding of the importance of data, actively developing a robust data culture.
Furthermore, it is crucial for individuals to take accountability and responsibility for the data within their respective areas, particularly with key data stakeholders.
Building Stakeholder Engagement
The approach you take in identifying and involving stakeholders depends on your organisation’s culture, maturity level, and specific data challenges.
Here are a few strategies to consider:
Problem-driven approach
Identify stakeholders facing data-related challenges. Conduct interviews or focus groups in key areas, particularly those heavily reliant on data (e.g. Business Intelligence/Data Analytics). Understanding their issues gives you insights into specific problems Data Governance can address. This approach aids in building a case for sponsorship by demonstrating the tangible benefits of governance.
Network-driven approach
Leverage personal connections and referrals to expand stakeholder interaction. Start by interviewing individuals with a deep understanding of the data landscape (and their role within it). Extend your network of stakeholders by seeking recommendations for other key players, ensuring a thorough exploration of the organisation’s data culture.
Organisational chart approach
Identify various business areas and their stakeholders by navigating the organisational chart. Choose representatives from each area for interviews to ensure diverse representation. While this method helps cover all business areas and prevent overlooking crucial stakeholders, it may demand more effort to initially engage individuals without direct personal connections.
Combined approach
Begin by interviewing stakeholders facing data challenges, then utilise the organisational chart to identify additional stakeholders across different areas. This method provides a balance between personal connections and comprehensive organisational coverage. Keeping thorough records of interactions facilitates progress tracking, dependency identification, and a thorough understanding of data flow.
Practical Advice for Stakeholder Collaboration
Regardless of your stakeholder engagement approach, ongoing communication and awarenessbuilding are essential.
You will need to regularly update stakeholders on the progress of the Data Governance journey and the insights gained through interviews and assessments.
By fostering open communication channels and demonstrating the value of Data Governance, you can increase engagement and create a shared understanding of the importance of data across your organisation.
While talking to your stakeholders, document data awareness gaps and training needs. This information will inform the creation of targeted training materials to address specific needs.
Providing training resources in advance can help prepare stakeholders and improve their understanding of Data Governance concepts and practices. Remember to keep engaging and communicating with your stakeholders as you progress through your Data Governance journey.
Education and Training
Communication is a key pillar of your Data Governance initiative.
Here are some considerations when developing your communication strategy:
Audience-focused approach
Tailor communication to the needs and interests of your audience. Consider their current level of data literacy, their roles within the organisation, and their stake in the Data Governance journey. This will help you deliver information in a way that resonates.
Transparency
Foster a culture of transparency and openness in your communications. Share successes and challenges. Be honest about the progress and outcomes of the Data Governance initiative. Encourage feedback from stakeholders and actively listen to their questions and suggestions. Two-way communication creates a sense of ownership and involvement among stakeholders.
Learning and improvement
Share lessons learned and best practices. Showcase the impact of Data Governance through stories. By sharing insights, you will foster a learning culture and encourage stakeholders to embrace Data Governance.
Timeliness
Communicate regularly and proactively throughout your Data Governance initiative. Keep stakeholders informed about upcoming activities, progress updates, and milestones. Timely communication helps maintain engagement, manages expectations, and builds trust.
Accessibility
Ensure that your communications are easily accessible to all stakeholders. Use a variety of communication channels, such as email, newsletters, intranet portals, and team meetings, to reach different audiences effectively. We’ve even seen cartoons and music being used to help bring Data Governance to life. Always make sure the information is presented in a clear and understandable manner, avoiding excessive technical jargon.
Change readiness
Use communication and information to address concerns and resistance, preparing the ground for change. Articulate the benefits of implementing Data Governance and the impact it brings to people’s roles.
Effective communication is an ongoing process integrated into every stage of your Data Governance initiative. Regularly assess communication effectiveness and adjust to stakeholder feedback and evolving needs.
Chances are Data Governance is an alien concept to your organisation, so you will need to build awareness and skills through continuous education and training.
Here are some considerations to keep in mind when planning and implementing your activities:
Grouping different sets of people:
Grouping people based on their roles and responsibilities is a practical approach to tailor the training content to their specific needs. Identify different groups, such as data stewards, data owners, and general data users, and determine the skills and knowledge expected from each group.
Soft and hard skills:
Soft skills, such as collaboration, communication, and change readiness, are essential for effective Data Governance. Hard skills encompass specific technical competencies, such as data analysis tools and understanding data definitions.
Awareness and education:
Awareness education ensures that everyone understands your Data Governance initiative, terminology, and its benefits to the organisation. Regular communication, focused sessions with key stakeholders, and change readiness training create awareness and preparedness for any changes.
General training:
Focus on providing training that incrementally lifts your organisation’s overall maturity. The training should cover core knowledge and best practices in managing data. Consider integrating this training with other annual training programmes to reinforce the importance of Data Governance.
Skills training:
Provide targeted training to enhance the skills required to use data effectively. We’ve seen the fallacy of adopting complex tools which only the most technically advanced can use. Therefore, if you plan to implement an advanced tool, you may need to look at more advanced training on analytics tools and data analysis techniques to ensure your people understand data definitions and lineage more deeply. It goes without saying that the training should align with the tools and technologies used within your organisation.
Role holder training:
For people with formal roles within the governance structure, provide training to support them in carrying out their responsibilities effectively. This training should cover collaboration skills, data issue management processes, root cause analysis, and clear guidance on their accountabilities and responsibilities. Emphasise the interconnectedness of roles within the organisation’s Data Governance structure.
Training delivery and timing:
Plan the timing and delivery of training programs strategically. Start with awareness education to prepare the organisation for change, and then gradually expand into other training programs as the Data Governance journey progresses. Provide content and guidance to role holders before their formal appointment, enabling them to understand the expectations and requirements of their roles.
Remember that effective communication and engagement are key to building capabilities and showcasing the benefits of good governance.
Continuously assess the effectiveness of your training programme, making adjustments to ensure continuous improvement.
Defining roles and responsibilities
Defining Data Governance roles and responsibilities is essential to ensure data is managed effectively, securely, and aligned with your organisational goals.
Effective role assignment creates accountability, promoting a culture of data responsibility and compliance.
Here are some common types of alignment with pros and cons to consider:
Alignment | Pros | Cons |
---|---|---|
Data Domain (e.g. People, Customer, Asset, Financial, Regulatory) |
|
|
Functional (e.g. Human Resources, Sales, Marketing, Finance, Risk & Compliance) |
|
|
Hybrid (e.g. Operations which can be a mix of functional and data domains) |
|
|
Business Process (e.g. Tender Process, Engineering Change Request Process) |
|
|
Systems (e.g. Data Warehouse, ERP system, Maintenance system) |
|
|
When determining the alignment of roles, consider the structure and culture of your organisation as it relates to your data landscape. Involve the HR function to discuss implications for job descriptions, responsibilities, and reporting lines.
Regular communication and collaboration among role-holders is key to successful Data Governance.
Example roles within a Data Governance framework:
Direction | Execution | |
---|---|---|
Role | Data Owner | Data Stewards |
What |
|
|
Who | Head of function | Data SME |
For | Each aligned area. | Each aligned area or sub area. |
Activities |
|
|
Assigning roles requires careful consideration of not only the seniority of a role holder but also their Data Governance mindset and related skillset.
Mindset
- Executive and senior leaders champion and own Data Quality as a critical indicator of performance.
- Data Governance is seen as a core pillar of success.
- All colleagues recognise the importance of data and contribute to its value creation.
- Leaders push for new projects to build in data management by design.
- Passionate about developing a Data Governance community and data-centric culture.
Skillset
- Data creators have the skills to define and implement data standards.
- People have the knowledge to use tools and technologies to manage data and its quality.
- Training is designed to give people role-based skills and capabilities to successfully drive Data Quality.
- People have the knowledge and skills to follow business processes ensuring accurate data capture and usage.
Defining your data
Data definitions and standards are the guidelines and specifications that define how data should be structured, formatted, and handled within your organisation.
They ensure data consistency, accuracy, and interoperability across different systems, processes, and applications.
Data standards can span many aspects of data management, such as naming conventions, data formats, coding schemes, and data classifications.
To begin defining your data, you need to start by understanding where data exists within your organisation. It is best to start with a single part of your organisation or a single set of data.
- Identify any existing business terms in use.
- Identify the types and data stored and any classifications if available.
- Link the data classification with the data attributes.
- List out the data attributes.
- Collate the data specifications for the data attributes.
- Where there are available documented sources – validate their use and use them where applicable.
- Understand the relationships between the source(s) and the data to be analysed.
Any available data artefacts may support this phase of work, as they provide the documented definitions. Ensure that any analysis undertaken is to the level required to expose the processes relevant to the business issue.
Sharing and communicating definitions
As you engage with stakeholders and build your Data Governance foundations, creating and populating key documents will help facilitate effective data management and decision-making.
Here are some essential artifacts to consider:
Business Glossary:
Develop a comprehensive business glossary that defines key terms and concepts used in your organisation. This helps standardise terminology, align
understanding, and reduce confusion or misinterpretation of data. It fosters collaboration and ensures consistent interpretation of data across teams
and departments.
Data Dictionary:
Develop a comprehensive data dictionary that defines key data and calculations used in your organisation. This helps standardise calculations and repeatable data creation. It fosters collaboration and ensures consistent usage of data across teams and departments.
Data Standards:
Compile and consolidate existing data rules, standards, and guidelines within your organisation. Evaluate their effectiveness, identify gaps, and determine if they adequately support data usage throughout its lifecycle. This serves as a reference for Data Quality and consistency and supports decision-making regarding Data Governance policies and practices.
By creating and populating these artefacts, you establish a foundation for effective Data Governance, promote collaboration, standardise understanding, and enable targeted actions to address data-related challenges within your organisation.
Assess, improve and monitor data quality
Understanding data criticality
Assigning criticality levels to data is a valuable approach for prioritising data improvement efforts and ensuring appropriate levels of quality based on the data’s importance and associated risks.
The following levels of criticality can serve as an example to help assess and manage data criticality within your organisation:

Data with a very high criticality exposes the organisation to significant consequences when it is inaccurate.
This encompass data that is made public and in which the public and the press have significant interest for example Sensitive / Personally Identifiable Information.
The impact of this data being inaccurate would have significant reputational damage.

High criticality data covers all data which is used to make critical board decisions and any data covered by, or reported to regulators, including Personal Identifiable Information.
The impact of this data being inaccurate is significant, and this data needs to meet a high standard, but issues with its quality do not have the reputational effect of very high criticality data.

Data with medium criticality is used for daily operations.
This data still needs to meet high-standards, but risks associated with issues are lower.
With this type of data there is often a need to balance more quality dimensions to ensure it meets levels required for trustworthy usage.

Low criticality data provides business benefits but making the data quality high does not add more value to the data.
This type of data is mostly used for infrequent operating purposes rather than decision making.
With this type of data “Timeliness” will often need to be prioritised over other quality dimensions.
By assigning criticality levels to your data, organisations can make informed decisions regarding the allocation of resources, prioritise data improvement initiatives, and focus on the appropriate Data Quality dimensions that are most relevant to each criticality level. This approach ensures that efforts are directed where they are most needed and where the potential risks and value associated with the data are the greatest.
Mapping the data lifecycle
Understanding how data moves through your organisation ensures the appropriate controls and standards can be applied at the right time.
An example could be the lifecycle of customer data from “Business Development” to “After-sales”.
A typical data lifecycle will look like this:
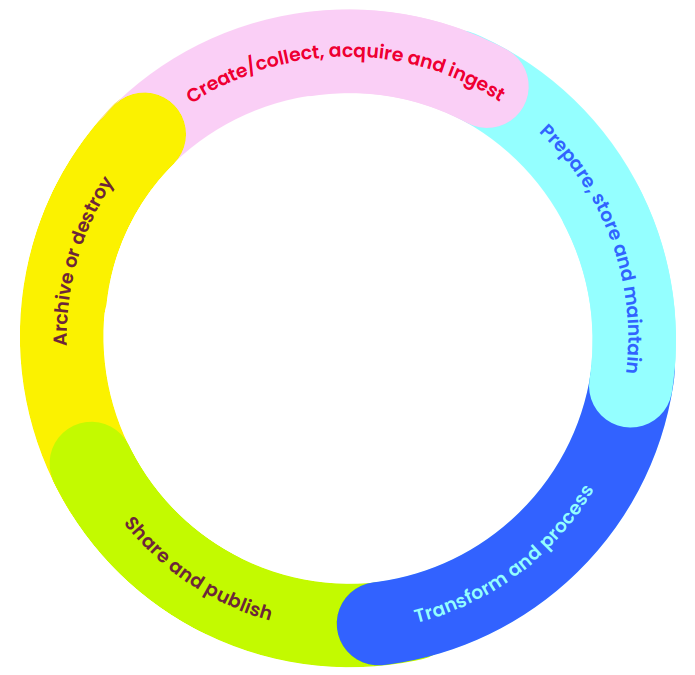
Here is a breakdown of the stages and their associated activities:
Create, collect, acquire and ingest:
This stage involves the creation or collection of data, either internally or from external sources. The focus is to ensure that the data meets the required standards and is fit for its intended purpose. This may involve data validation, data capture guidelines and Data Quality checks during the acquisition process. The acquired or collected data is ingested into the organisation’s systems or data repositories. It is important to maintain data integrity during the ingestion process, including data cleansing, data mapping and data transformation if necessary.
Prepare, store and maintain:
Once data is ingested, it needs to be prepared, stored, and maintained in a controlled and organised manner. This includes activities such as data profiling, data integration, data storage optimisation, data security measures and maintaining metadata about the data.
Transform and process:
This stage involves transforming and processing the data to make it usable for various purposes. It includes activities such as data cleansing, data enrichment, data integration, data aggregation and applying business rules or calculations. Clear documentation of the transformations and calculations is important for transparency and data lineage.
Share and publish:
Data should be shared and published in a way that is accessible and understandable to the intended users. This stage involves providing access to glossaries, dictionaries, and metadata to help users interpret and utilise the data effectively. Quality metrics and information about data sources should also be made available to support informed decision-making.
Archive or destroy:
Data retention and disposal policies should be established to determine when data should be archived or destroyed. Clear documentation and adherence to regulatory considerations are essential in this stage to ensure data is retained or disposed of appropriately.
When progressing through these stages, it is still vital to maintain Data Governance principles; this includes Data Quality monitoring, Data Lineage tracking and Continuous Improvement.
Communication and collaboration across different roles and stakeholders involved in each stage are crucial for effective Data Governance. A Change Management Process will make sure changes to data requirements are reviewed and approved by, as well as communicated to, the appropriate teams for implementation.
Data quality management
Earlier, we highlighted that a key business driver to embed Data Governance is to make sure that the data quality in the organisation is fit for purpose.
Data Quality rules are required, but it is unlikely that one set of rules will apply across all your data sets. Since different types of data will serve different purposes, it is important to consider data quality metrics specific to your dataset`s purpose.
Balancing the quality metrics applied to data is crucial for effective Data Governance.
Here’s a summary of the key quality dimensions (or data quality measurements) and their significance:
Accuracy (e.g. Telephone number)
Determines if the recorded value aligns with the actual value. Data accuracy is essential for making informed decisions and avoiding errors or misinterpretations.
Completeness (e.g. Email missing on customer records)
Examines if any expected data elements are missing. Complete data provides a comprehensive view and supports accurate analysis and reporting.
Auditability (e.g. Errors can be traced back to root cause)
Considers if the data flow is welldocumented to identify potential issues throughout its lifecycle. Auditability ensures traceability, accountability and the ability to investigate data-related problems.
Consistency (e.g. First name and surname in the right order)
Checks for discrepancies or conflicting values within the data. Consistent data ensures reliable and coherent information across different sources or systems.
Timeliness (e.g. Customer billing address is up-to-date.)
Assesses whether the data is up-to-date and arriving within the required timeframe. Timely data is crucial for making real-time or time-sensitive decisions.
Validity (e.g. Date of birth format matches business requirement)
Verifies if the data adheres to the defined business rules and expectations in terms of format and value. Valid data is reliable and suitable for its intended use.
When building the foundations of good Data Governance, it’s important to have a prioritised approach to understanding, documenting, monitoring, and improving Data Quality because the scale of the challenge can be daunting.
By leveraging the criticality framework introduced earlier, you can prioritise areas that require higher Data Quality levels based on business needs and benefits. With a focus on the appropriate Data Quality dimensions and realistic metrics, you can raise Data Quality to meet the varied needs of your stakeholders and use cases.
Prioritised data quality allows for a more targeted and efficient approach to Data Governance, ensuring your data delivers better decision-making, operational efficiency and customer satisfaction.
Your first Data Quality assessment will likely focus on your organisation’s key issues. As organisation maturity develops, you should aim to create a repeatable assessment of the quality of your data using a combination of the above metrics and your data standards.
As your Data Quality process discovers issues, your next task will be to log the most serious issues via a central register.
Building the Data Issue Register
A Data Issue Register provides a coordinated method of documenting and tracking your organisation’s most serious data issues.
Data-related problems, inconsistencies, errors, and concerns are logged, monitored, and managed via a central repository that ensures transparency, accountability, and effective resolution of data issues throughout their lifecycle.
Here are some key elements of a Data Issue Register:
Issue Identification
- Description: A clear and concise description of the data issue.
- Source: Indicating the issue’s origin (e.g., specific data source, process, application).
Classification
- Type: Categorisation of the issue (e.g., data quality, accuracy, completeness, timeliness).
- Severity: Evaluation of the impact or severity of the issue on business operations.
Metadata
- Date Detected: The date the issue was first identified.
- Detected By: Person or team responsible for identifying the issue.
Status Tracking
- Current Status: The current state of the issue (e.g., open, in progress, resolved).
- Assigned To: The individual or team responsible for resolving the issue.
- Target Resolution Date: The expected or agreed-upon date for resolving the issue.
Root Cause Analysis
- Investigation Notes: Detailed information about the steps taken to analyse and understand the root cause of the data issue.
Resolution Details
- Corrective Actions: Specific steps or measures taken to address and resolve the data issue.
- Verification Steps: Procedures to confirm that the resolution is effective and the issue is fully resolved.
Communication Log
- Updates: Records of any communications, updates, or discussions related to the data issue.
- Notifications: Information about any notifications sent to stakeholders regarding the issue and its resolution.
Audit Trail
- History: A chronological record of changes to the issue status, resolutions, and other relevant details.
Governance and Compliance
- Compliance Requirements: Indicating whether the resolution aligns with regulatory or organisational compliance standards.
- Documentation: Links or references to relevant policies, procedures, or guidelines.
Reporting and Analysis
- Metrics: Key performance indicators (KPIs) related to issue resolution and data quality improvement.
- Trends: Analysis of recurring issues or patterns that may indicate underlying systemic problems.
The issue register helps prioritise and remediate data issues, quantify the need for Data Governance investment and identify areas that require immediate attention. It provides insights into common pain points and informs decision-making regarding resource allocation and improvement initiatives. The register also allows you to document who is responsible and accountable for resolving data issues.
Once you have a prioritised list of issues, focus on root cause analysis to resolve the issue at source rather than downstream. With data owner support, ensure staff have the right data, people, process and technology skills to resolve the causes of the most important issues.
Finally, by leveraging your standards and metrics for data quality, you can build a repeatable assessment and monitoring capability that can instantly report and track future data quality issues.
Technology and Tooling
Data Governance Tooling
Do you need a Data Governance tool?
Once you have created the Data Governance fundamentals, you can explore how Data Governance technology could extend your foundational gains.
Here are some useful pointers when considering tooling options:
Define the purpose
Gather a clear consensus on the challenges and goals you wish to address with a Data Governance tool. This clarity will guide your selection process and help you find a tool that aligns with your objectives.
User engagement and experience
Involve end users in the selection process and ensure engagement through the implementation. Consider how they need to interact with the tool. The tool should be user-friendly and intuitive, providing a seamless experience to encourage adoption.
Stakeholder engagement
Look for a tool that facilitates collaboration and engagement among stakeholders. It should allow for efficient communication, feedback, and knowledge sharing, enhancing the overall Data Governance practices within your organisation.
Move beyond storage
Avoid treating the tool as a storage solution. While some Data Governance tools contain business glossaries and data dictionaries, the benefit should go beyond storage. Look for a tool that enables collaboration, engagement, and active use of the data and resources it contains, and ensure it integrates to your system architecture and not siloed.
Ease of use
The tool should be easier to use than alternative methods of managing Data Governance. It should simplify tasks, streamline processes, and make it easier for stakeholders to contribute, access, and derive value from the information within the tool.
Value-driven solution
Assess how the tool can provide tangible value. Consider how it can support decisionmaking, improve data understanding, streamline processes, enhance Data Quality, and contribute to overall Data Governance maturity.
By focusing on these aspects, you can select a Data Governance tool that not only meets your needs but also empowers users, fosters collaboration, and adds value to your Data Governance initiatives.
How should the technology work with your people and culture?
Data Governance tools can’t replace the people element of effective Data Governance – the key is to use technology to enhance the capabilities and performance of existing data stakeholders, knowledge workers and processes.
Here are some key points to consider:
People capabilities
The success of a Data Governance tool relies on having a network of people with a deep understanding of data and its governance. Ensure you have the right people in roles and provide adequate training to build the necessary knowledge and capabilities to use the tool effectively.
Organisational culture
Evaluate if your organisational culture is ready to support the use of a Data Governance tool. The tool requires active engagement and participation from users across the organisation. If the culture is not receptive to using technology for Data Governance or lacks the necessary data literacy, it may hinder the tool’s effectiveness.
Start with familiar tools
In the early stages of your Data Governance journey, consider using readily available productivity tools to document information about data. This allows for a simpler and more familiar approach, especially when the skills and knowledge of key users are still developing. Gradually, as you scale your Data Governance capability and identify the need for more advanced functions, the need for a dedicated Data Governance tool may become apparent.
Meaningful information and value
Remember that simply gathering vast amounts of information into the tool should not be the focus. The tool should enable the provision of meaningful, timely, accurate and useful information about your data across the organisation. It should facilitate data understanding, decision-making and support the overall Data Governance journey.
Ultimately, Data Governance tools should align with your organisation’s specific needs and readiness. They should complement your Data Governance efforts and empower users to manage and exploit data as an asset for growth and success.
Remember that what’s right for one organisation may not be right for you. By discovering current and future use cases, you can build a transparent, unbiased assessment of the tool’s capabilities against your own selection criteria.
Game changer: Generative AI shaking the Data Governance world
Generative AI is here to stay. Whether you are already investing in Gen AI or still exploring your options, it is a game changer for how business manage their data assets.
Challenges for businesses
Generative AI introduces unique challenges that demand vigilance at input, processing and output. With the introduction of AI regulations and laws, there is no getting around that this technology is more ‘demanding’ than other traditional data solutions, and as such will require more complex governance.
- The ‘black box’ concept makes governance tricky! We might know what the AI is ingesting and producing as output or predictions but we don’t know the “why” of its predictions. This makes traceability way more complicated.
- It brings with it increased data documentation to teach context and accuracy while keeping with privacy and security requirements.
- And getting concrete value out of AI and GenAI is very dependent of asking the right business questions and building the right prompts.
The holy grail is good data. Or else, your Gen AI will be contextless without structured prompting and will be EXACTLY representative of your data – you need to be ready.
AI makes your data speak to you – Data Governance makes it say the right thing
Your AI-powered solutions will be ingesting the data, making decisions and acting autonomously to achieve specific objectives. For minimal business disruption, this ideally needs to be seamlessly embedded in your day-to-day processes.
AI agents are software programs or entities designed to do exactly that. These agents can vary widely in complexity, from simple rule-based systems to sophisticated machine learning algorithms.
The core idea of agents is to use a language model to choose a sequence of actions to take – the language model is used as a reasoning engine to determine which actions to take and in which order; not very different to human reasoning. The eureka moment comes when we give the agents the tools to act. Good data is non-negotiable.
A robust Data Governance framework is your guiding light. It’s the unsung hero that ensures that your AI-powered agents will ACCURATELY understand your prompts, ingest the CORRECT data, in the right format and make the RIGHT decisions or predictions.
How does a Data Governance framework make your AI journey seamless? It’s all about considerations – data quality, ‘human’ roles and responsibilities, education, monitoring and compliance – which allows for trust, ethical use and bias removal.
Starter for ten checklist:
- Understand your business landscape for Gen AI improvement.
- Map your information flow and data dependencies.
- Transform your Data Strategy from a document to action.
- Elevate your data platforms to meet Gen AI standards.
- Uncover and address biases lurking in your data.
- Assess the quality of data powering your Gen AI models.
What challenges will you need to overcome?
Addressing challenges while implementing your Data Governance journey is crucial to ensuring a successful outcome.
Here are some strategies to tackle common challenges:
Lack of awareness:
Despite regular communications, some of your people may still be unaware of Data Governance activities due to organisational silos. To address this, have easily accessible and understandable material that clearly communicates the why, how, and what of Data Governance. This material can be readily shared to provide instant information and raise awareness about the importance and benefits of Data Governance.
Existing systems and processes:
During your data landscape analysis, you may encounter systems and processes that pose challenges to your Data Governance implementation. If attempting to solve issues in these areas would create more problems, work closely with the affected teams to identify pain points and explore alternative solutions. Collaboration and open communication will be essential in finding viable approaches.
Difficult stakeholders:
Dealing with stakeholders resistant to change requires a focus on active listening and responsiveness. Take the time to understand their concerns and address them effectively. Additionally, connect them with other people involved in different parts of the data lifecycle who can provide firsthand experiences and demonstrate the need for change. This humanises the process and fosters a sense of collaboration.
Tools as solutions:
It can be tempting to bring in a tool or start a Gen AI program to solve your Data Governance problems, but without the right foundations these can hinder rather than help. A tool needs people with the skills to use it across your organisation; otherwise the tool will end up only being added to and used by a select few. You also need to select the tool based on specific use cases, which is easier to do once Data Governance foundations are built. For Gen AI, if you have poor data quality or siloed data you will not get the best out of it and could end up making the wrong decisions. Ultimately tools should be enablers not solutions in your data governance journey.
Historic failures:
Past unsuccessful attempts at Data Governance can lead to apathy and scepticism among stakeholders. Address this directly by acknowledging the previous failures, emphasising the continued need for Data Governance, and explaining why the current implementation is different and more likely to succeed.
Lack of sponsorship:
Data Governance is a change management endeavour. Having a sponsor who is at a very senior position helps the programme get traction where things might stall. They do not necessarily actively get involved in the delivery of the Data Governance programme, but they act as a leadership authority who champion data governance and own the budget for its implementation.
Learn from past mistakes and clearly articulate the changes and improvements in the current approach. Maintain open channels for feedback, listen actively, and be prepared to pivot if necessary, based on stakeholder input.
By proactively addressing these challenges and tailoring communication and engagement strategies, you can help overcome resistance and create a more receptive environment for your Data Governance initiatives.
How can Oakland help?
Wherever you are on your Data Governance journey, Oakland can help you make the next step.
We offer a range of custom services spanning the entire Data Governance lifecycle. You can choose to engage us at specific points on your journey, or we can partner you through the entire process of defining and implementing Data Governance.
A – Data Ownership Design
Have you ever noticed how people can be sensitive about their prized possessions being tinkered with? Owning something can make you feel really protective of it, especially when it’s an asset that increases in value over time.
At Oakland, we have distilled the collective knowledge from our experts and in depth industry knowledge into a rounded service offering to help you design and build an Ownership model to suit your organisation This ensures data accountabilities and responsibilities are in place from day one to drive a data-centric culture.
This is a key aspect of Data Ownership – if someone is accountable and responsible for a piece of data, they’ll ensure that it is defined accurately, maintained correctly, and used responsibly. More importantly, there’s a go-to person or team that can be approached with questions or changes that need to be made to the data. Ownership means accountability. By implementing an Ownership model for data within your organisation you create a focus on responsibilities and accountabilities that support a value-creating data culture.
B – Data Governance Maturity Assessment
Most organisations recognise the value of more general governance and this translates directly into Data Governance. Data Governance should help you manage your data more efficiently and effectively, instil an ethos of data ownership and stewardship, and progressively work to improve the quality and reduce the risks surrounding data and its use.
Our Data Governance Maturity Assessment reviews the state of your current organisational Data Governance against a detailed framework of industry standards to understand strengths and weaknesses, and help you decide where to target your improvement efforts.
C – Data Quality Assessment
Some people would say that trust is everything. Data is a business asset that needs to be trusted for an organisation to use it effectively. But, not all data is equal and, ultimately, all data will have its trustworthiness questioned. Failing the test can be expensive, either measured in organisational outcomes or in terms of duplicating or repeating of effort.
Our Data Quality Assessment provides a framework for assessing your data in terms of its completeness, accuracy, consistency, integrity, timeliness and auditability. All the essential benchmarks of Data Quality.
It will provide recommendations on the appropriate Data Quality framework(s) to fix issues and suggest the right Data Quality business rules and thresholds.
We will talk you through the assessment results to help you fix the issues identified and help you move forward.