Digital Twins – A longer history than you may imagine.
The notion of digital twins is nearly 20 years old. American academic Michael Grieves coined the term at the University of Michigan in 2002 when he was considering product lifecycle management. He proposed that a digital model of a physical system (for example, a car) could be created as a virtual entity containing information about the physical system and be linked with the physical system through its entire lifecycle. Data could then flow between the real and virtual spaces to keep the twins synchronised.
The idea of a creating a twin of a physical system has a longer history. Famously, when disaster struck NASA’s Apollo 13 spacecraft in 1970, engineers on earth were able to use an earth based physical twin to test possible technical solutions, with the chosen option implemented, enabling the spacecraft and its crew to safely return.
Today, digital twin thinking, along with new technologies including the Internet of Things (IoT) and high bandwidth telecommunications, is providing sophisticated insights. In Formula 1 racing, for example, Lewis Hamilton’s Mercedes F1 has over 200 sensors connected in real-time to pitlane telemetry providing over 300GB of performance data during a race to the team’s pit crew and the car’s digital twin.
Digital twins are models used to understand, predict, and/or optimise performance. However, not all design or analysis models are digital twins. In my opinion a digital twin needs condition information which is specific to the system and updated during operation.
Image a bank of four identical gas turbine generators. Initially all would behave very similarly, but over time, each would experience different conditions: when they were turned on, and off, how quickly they were spun-up; how hard they were driven; were they used during the hottest or coldest days. Each turbine has had a different life, and each will have its own digital twin fed by its operational data. These individual digital twins can be used to inform maintenance schedules or operating policy.
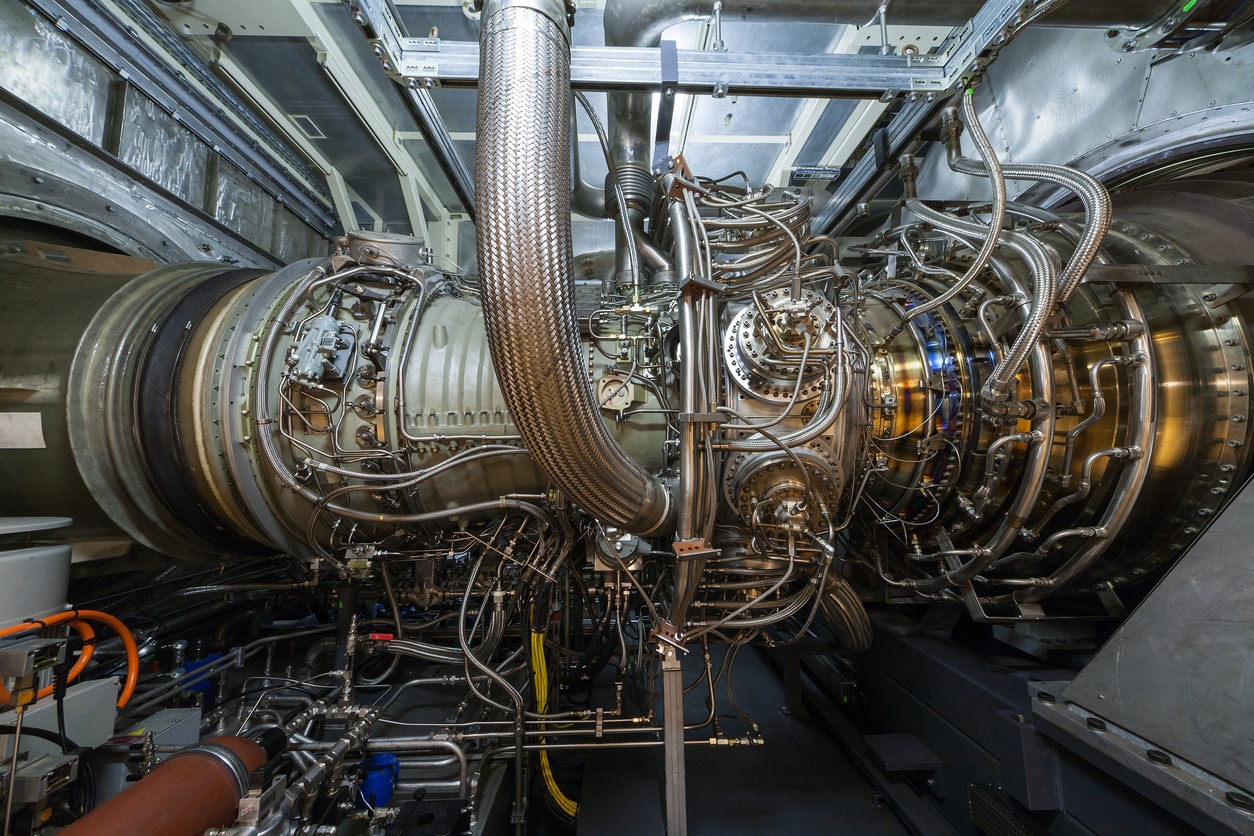
Cogeneration plant gas turbine engine
Digital twins are invaluable for performing ‘what if’ analyses, to assess new operating parameters or design ideas to be tested. When connected to IoT sensors, digital twins ingest operational data. This allows performance monitoring which can inform maintenance schedules, or feed into the design of future systems. Some of Oakland’s clients are already developing capabilities; Yorkshire Water, for example, aims to create a digital twin of its asset base in the west Sheffield area by combining data from its acoustic, flow, pressure and water quality monitors (read this WWT new item).
Digital Twins in infrastructure
The digital twin concept has also begun to gain traction in the UK architecture, engineering and construction sector, particularly after the National Infrastructure Commission published “Data for the Public Good” in December 2017. Around a year later, the Centre for Digital Built Britain published the Gemini Principles (defining core elements for both single instances and the ‘national Digital Twin’). A Digital Twin Hub was subsequently launched to bring together major asset owner-operators and agree some common principles.
Digital twins can be applied to a very wide range of systems, not just infrastructure projects. I have seen digital twins for gas turbine engines and have proposed a digital twin for an on-line investment bank.
Mathematical models and data models
This is a little contentious, but I want to make a differentiation between what, for these purposes, I will call ‘mathematical models’ and ‘data models’. And before the Twitter storm erupts, yes, I know life is not so simple, as all models contain both maths and data. I could use other terms such as ‘classical’ and ‘modern’, but there are similar issues. My point is that models at either end of this maths and data spectrum have some specific characteristics, that enable them to play slightly different roles in digital twins.
By ‘mathematical models’ I think of predominantly physical systems where an understanding of how the target of the digital twin operates is codified with governing equations. For example, 20 or more years ago, I was building ‘mathematical models’ using Green’s theorem to solve Maxwell’s equations to understand how radar bounces off aircraft. The equations were known, the model merely enabled a computer to solve them for the complex geometry of an aircraft. With infrastructure projects, such models might involve knowledge of geology, of concrete structures, and of corrosion. These behaviours are combined into a model, which can answer questions such as how much load can added before cracks appear, or in 20 years’ time what would the residual strength be? Of course, these ‘mathematical models’ can be applied to non-physical systems; we just need to understand the behaviours (for the on-line investment bank, for example, we can derive relationships between customers and products, and between customer service and retention).
These mathematical models are powerful but to count as digital twins they need to include system-specific operation or historic data. ‘Data models’ use data science to determine the complex and subtle ways the streams of data inputted influence the outcomes. The skill of the data scientist is to understand not just the data available but crucially to understand the questions being asked. With this the model can be honed to uncover the relationships and insights.
Digital twins and data science
In a traditional IoT-connected example, operational data from the physical asset is connected to the digital twin. This will involve data engineering but is unlikely to include data science. Things get more interesting when data science is also deployed. A data model can learn the actual behaviours of the system, in a way the ‘mathematical model’ cannot. This enables unusual operation to be quickly identified, and for failures to be predicted.
Data models can be immensely powerful, Oakland Group’s Intelligent Forecasting Platform, for example, can forecast project outcomes 80% more accurately than project managers. This is based on years of project data being assessed. This is an example of a digital twin based on a data model: the model is derived from (trained on) historical data, and operational data feeds regularly update the model. In this case, the update frequency is days or weeks rather than the milliseconds one would see in the F1 example.
While data models are well suited to digital twins, they may struggle if there is a step change in the twinned system. For example, if an organisation makes a wholesale change in their project management policy, procedures and operations, the historical data on which the model is based may not reflect current operations. In this scenario, the data model can be used to support the development of a ‘mathematical model’, helping to determine the behaviours and provide validation. The ‘mathematical model’ can then address this step change in operations, while the ‘data model’ is trained on new data.
Some lessons for programme management
It is clear that digital twins are very powerful, but the term is often misused. A good definition is a model of a system that is updated with system-specific operational data. The twinned system can be physical and non-physical, and depending on information available and its application, the model can be a classical ‘mathematical’ model or a ‘data model’.
How can you gain benefit from digital twins? In theory, getting a digital twin is straightforward. The advent of IoT technologies means that gaining operational data from physical systems has never been simpler, and the rise of data models and cloud computing means many more systems can be modelled using this data. Lots of companies offer off-the-shelf solutions, but there are pitfalls. For example, systems can over-specify data leading to “a wood for the trees” issue – I have seen IoT systems specified with 1/10th second refreshes for systems where temperature changes occur over many minutes.
As with all modelling, the key question is: what do you want the twin to achieve? How will it connect with your business goals and add value? How do you link the data engineering with the desired operational excellence? Putting effort in to understand this will ensure time and money is not wasted on a poorly implemented digital twin. Better digital twins will highlight the practical value that can be delivered, avoiding the concept being derided as mere hype.
Author: Martin Pocock, Technical Delivery Lead at The Oakland Group